AI in Consumer Finance
Are we finally reaching the “plateau of productivity”, or are some of us lost in the trough of disillusionment? We’ll find out, as Michelle Katics, BankersLab CEO interviews John Curry, an AI practitioner in finance.
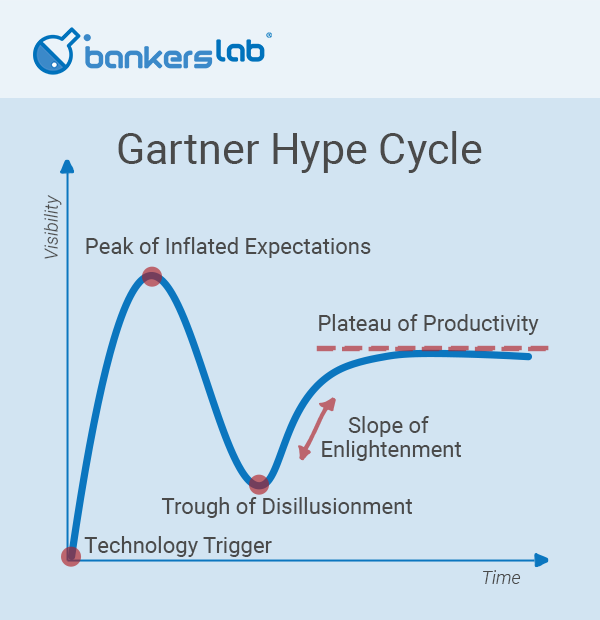
Michelle: I would like to introduce everybody to John Curry who has supported BankersLab as a faculty member in the area of machine learning and artificial intelligence. John, tell us about yourself and the work you do in the industry.
John: I’m a data scientist and a data science manager strategist for the last 10 years. I work hands-on building AI models and creating products, as well as advising companies in the financial sector. In recent years I’ve started to teach and train as well. I deal with complete newbies to the area through experienced data scientists. Seeing how AI has actually been used to create value, I learn as much from the students as they from myself.
Michelle: We’d like to understand where we are today in the financial sector with machine learning and AI. For example, think about the Gartner Hype cycle. Have we reached the plateau of productivity? Are we still in the trough of disillusionment? Where are we?
John: I wouldn’t say we’re anywhere near the plateau of productivity. If anything, I’d say we’re just turning the corner into the trough of disillusionment. So, there is plenty of enlightenment to come over the next five to 10 years.
Michelle: How many of the AI projects today are successful?
John: If I was to make a guesstimate, I’d say half of AI initiatives result in fully functioning projects. They are similar to really complex IT projects like ERP projects. There is a very, very high failure rate, and of those, maybe one in five actually makes some return on investment.
I think it’s been really, really tough the last 10 years. It is just a huge learning curve for everybody. I think in the last two to five years, businesses are making much more judicious bets.
Michelle: A failed test is still successful in terms of its learning. If four of five projects fail, how would you describe these four projects that you don’t think are bringing financial value?
John: There’s no question there’s learning value, and I think that failure rate is dropping and it’s going to continue to drop. Fifteen years ago, I don’t think there was the level of understanding in the business community as to what a good project looked like or how to approach it. Whereas, now I think everyone goes in with open eyes. Are we going to put it into production? How are we going to make a product or service side of this? Is the customer going to like it? The market is becoming much more educated, such as through the type of hands-on workshops that BankersLab delivers.
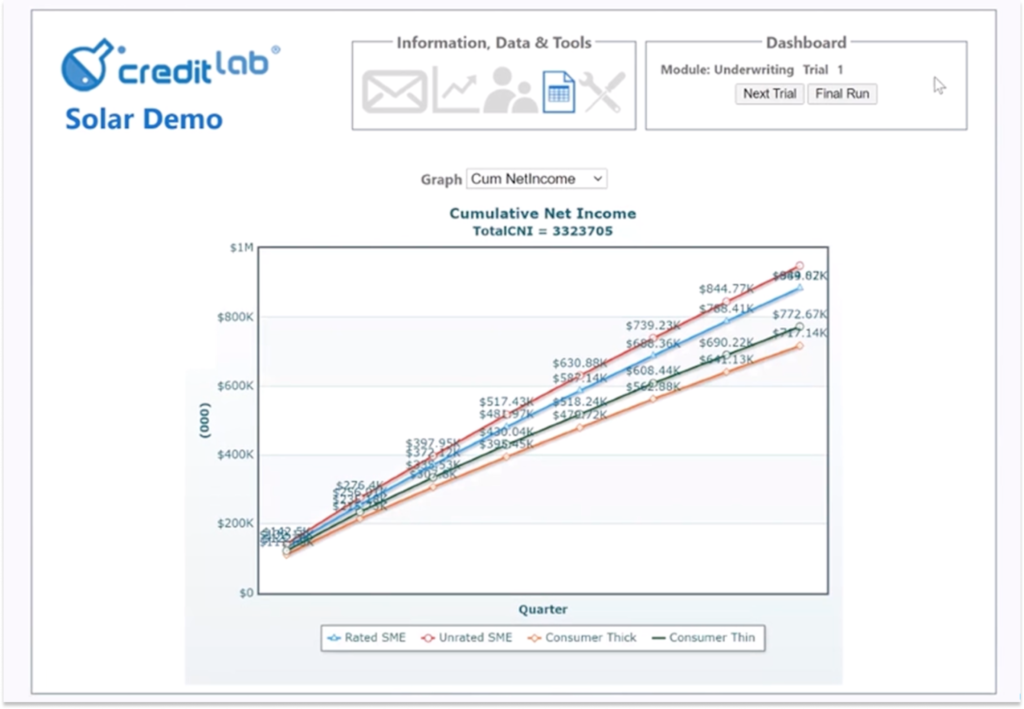
Michelle: Let’s talk about that one successful project. What does success look like?
John: There has been a lot of success. For example, in the Philippines, Telecom companies there started to collaborate with banks and with FinTech’s to start to bank the unbanked. You can combine all these very disparate pieces of information that on their own may not seem significant, but are helpful when looked at holistically. By combining mobile phone history and other
AI doesn’t really operate well in a silo. But if you put it together with the massive influx of data, including mobile device data and open banking data, you see some nice effects.
Michelle: Per the example you gave in the Philippines when you combine all these disparate data sources and you use your MIL toolkit, are you focusing more on just targeting people and then you send them through a normal credit approval process? Or are you also building the credit approval process from that mobile data alone?
John: In some cases the targeting has affected the approval process as well. Often you should think of these things all the way through the process. For example, if we’re going to do something with AI at the top of the funnel but if the customer is sent through the same approval process, it’s not going to work. Is a car just a horse and cart with an engine? It’s not really – it changes the game completely.
Michelle: So, lenders should rethink their product design at the same time? We’ve worked on that with our clients. Now that we have these new data sources, let’s step back and rethink not just the product offering, but to your point, the whole funnel – the whole process.
If you can select borrowers solely based on mobile data, but there’s no traditional data, and that’s your filter for approval, then you haven’t really gotten anywhere.
John: Big time.
Michelle: I think folks have a perception that machine learning and AI is complete rocket science and it’s hard to demystify. Are there any examples of a simple solution?
John: That happens a lot of the time. We might think we should use the latest cool model. That can actually be a hammer looking for a nail.
One example was on suspicious transaction alerts within a financial institution, so it’s basically text information. The traditional way of handling was pouring through this text and assigning them with green, orange or red. The proposal was to automatically classify and prioritize these risks. But there was an awful lot of resistance from the domain experts who felt that this might not be a transparent process and it might not work.
Once we had domain experts involved, we were able to identify key features which worked well. We came up with some hand-curated features and the result was actually a really simple model. It was a decision tree which is quite nice because you can visualize the result and understand the solution.
Michelle: Would you call the process that you’re describing data tagging?
John: Yes, it was tagging in relation to severity so it’s a little bit like sentiment analysis. We are looking for various phrases, words and combinations.
Michelle: The industry is complaining that they’re saving all these lakes of data and they don’t know what to do with it. he process you’re describing also applies to debt collection, for example, where you’ve got agents on the phone and you have voice to text data.
John: We aim to empower people to do their job better and to get rid of manual tasks that add no value. The more complex job of AI is chiming in with suggestions to the humans. To learn to interact productively with AI, even if you consider it a black box, is actually a skill in itself.
Michelle: As we try to pick up these skill sets and learn, what are some classic rookie mistakes that we should try to avoid?
John: First, just be led by the business problem, and continue to be razor-focused on that business problem. Then, just start out with some very simple method of solving that problem that may not involve AI.
Next, we need to asses:
- What are the costs?
- What are the challenges?
- What are the benefits?
Finally, consider AI alongside a simple solution and asses whether the AI is actually going to add serious value.This design process is a helpful exercise even if you don’t do the project. Consider how the AI is going to help you and establish your method of evaluation. Then you can understand whether the AI project is actually working or not.
As a result, we have this concept that machine learning of is a process of training and testing. You don’t want your model to cheat, and you want to train the model, but then give it a blind exam to make sure it’s working.
This training and testing is really, really important, and it’s actually an awful lot harder than it seems – especially in forecasting situations. Imagine I can see that this model predicted three months ahead. How do you know this forecast is going to generalize? The solution is to pilot things in a live situation and conduct your evaluation. The last mile is getting out getting out of the lab into the market.
Michelle: Last year, BankersLab began conducting courses in experimental design (A/B testing). Based on this discussion, it seems necessary for AI and machine learning. Are we up to the task?
John: It’s not just bankers, it’s difficult for all of us. If we just launched a product, we to immediately ask:
- Is our sample size big enough?
- Is there a confounding factor?
You need to get into that kind of analytical mindset. You can pick up the math of the A/B test in a day or two, and it’s been around for 100 years. We need the courage to do it and say it. You need to communicate with team members and management about the rigor in the process.
Michelle: Even a simple test that’s done well can be gold.
John: I think you hit the nail on the head. A simple task, simple model and a little program – done well, can have a much bigger impact than being able to say you used some type of AI that sounds fancy.
Michelle: Are we finally growing tired of the buzzwords and ready to just get down to business?
John: Yes!
Michelle: What are some of the biggest hurdles you see out there that people are encountering when they try to implement solutions?
John: I’d say it’s the communication gap between the different roles on these projects. We do have a problem with supervised learning with the amount of data needed for it, that that’s one technical issue, and there’s a lot of things in the pipeline which accept supervised learning. The tools and technologies have advanced in leaps and bounds.
We need to educate business people and explain the capabilities, and data scientists need to absorb domain knowledge.
Michelle: We’ve conducted a number of workshops which are basics of lending and digital banking for the technologists to ensure they understand the critical cause and effects within these lending portfolios. They don’t need to become the domain expert, but they need to be able to collaborate.
John: This collaboration is worth even more than the next technological breakthrough.
Michelle: Learn-tech.io has been offering AI for leaders to help them lead the change. They don’t need to start coding Python, but rather they need to provide leadership to identify the business problems.
John: A great business leader is very well versed in getting up to speed in new area very fast, whether it’s finance or IT data governance. AI is now a big part of that.
The BankersLab workshops help you get behind the headlines.
So let’s say we’ve now sparked some excitement among folks to do work on AI and Machine Learning. Do they need to go get a PhD? What kind of qualifications should they get?
John: There are a number of routes. Master of Science and PHD’s are definitely common enough – but I’ve also seen college dropouts and high school graduates doing well. With coding and AI you can show what you can do pretty quickly. There are many resources to self-learn.
Find work in your company to get practical skills, and you can join courses online where you can meet other people doing similar work.